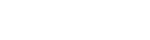
AI-Powered Fraud Detection
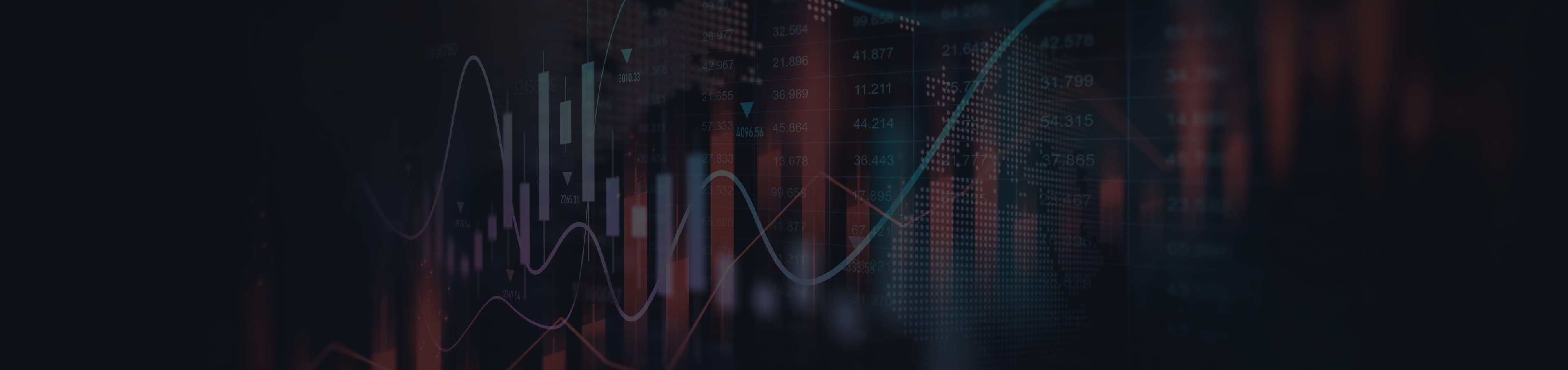
- Project: WolfShield
- Industry: Banking, Insurance, and Retail
- Challenge: Reducing investigative workload for human fraud detection panels
- Solution: AI-driven anomaly detection models with interactive visualization and alert management
Background
Fraud detection in financial transactions has historically relied on human investigators to manually review flagged transactions. While effective, this process is time-consuming, resource-intensive, and susceptible to human error. To enhance efficiency and accuracy, a cutting-edge AI-driven anomaly detection system was developed and deployed across multiple industries, including banking, insurance, and retail.
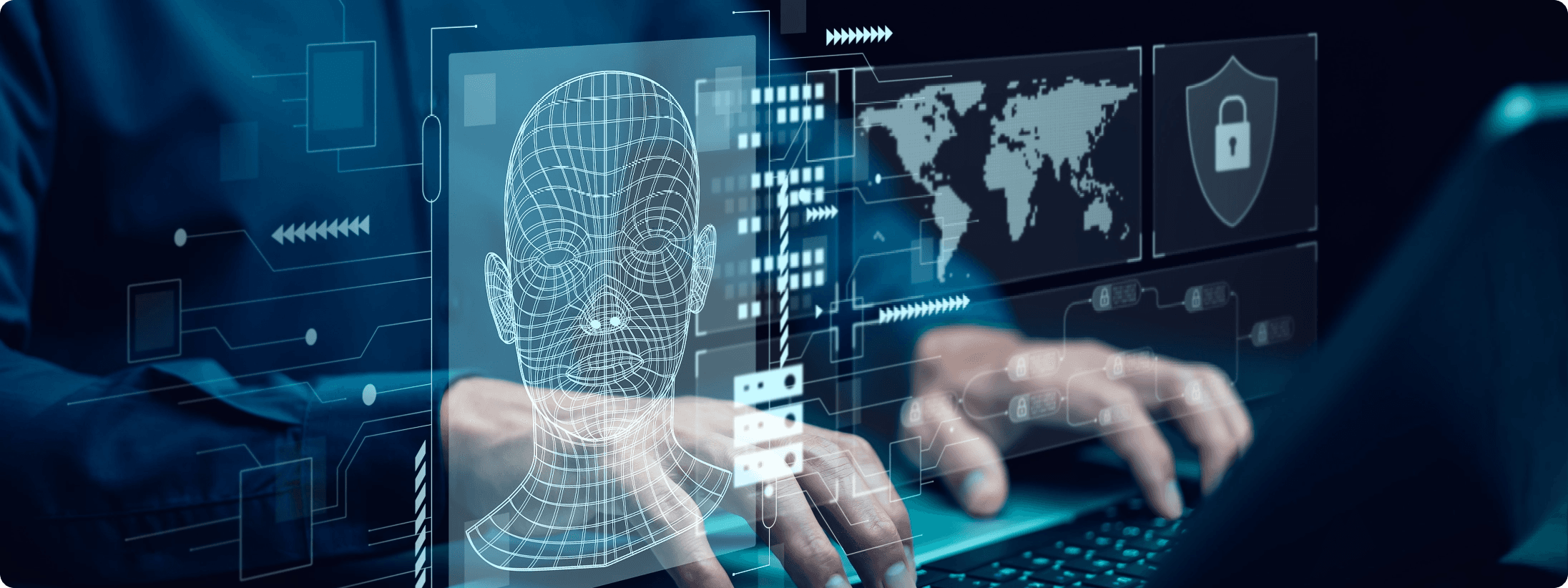
Objectives
- Automate the identification of potentially fraudulent transactions.
- Reduce the workload on human investigators by prioritizing high-risk cases.
- Enable each client to have a tailored model trained on their unique data.
- Provide an intuitive interface for clients to manage alert sensitivity.
- Offer interactive transaction visualization to enhance investigative insights.
Solution Implemented
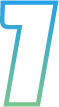
Custom Anomaly Detection Models
- Multiple anomaly detection models were trained and deployed for each client, ensuring a customized approach tailored to individual data patterns.
- The majority of models were developed using autoencoders, a deep learning technique effective for detecting deviations from normal transaction behavior.
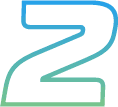
Adaptive Alert Management Interface
- Clients were provided with an interface to dynamically adjust alert thresholds, allowing them to fine-tune the sensitivity of fraud detection.
- This feature enabled organizations to balance efficiency and security, either reducing false positives or increasing case scrutiny as needed.
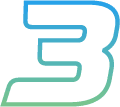
Interactive Transaction Visualization
- A novel feature allowed clients to interact with the bottleneck layer of the autoencoder, providing an intuitive visualization of transactions in relation to one another.
- Statistical overlays provided additional insights, allowing investigators to superimpose real-world risk factors onto transaction patterns.
Results & Impacts of AI Implementation
Efficiency Gains
Human investigators could now focus on the most high-risk transactions, improving resource allocation.
Accuracy Improvement
Fraud detection became more precise with fewer false positives and an enhanced ability to identify subtle fraud patterns.
Scalability
The modular nature of the models allowed seamless deployment across various financial institutions.
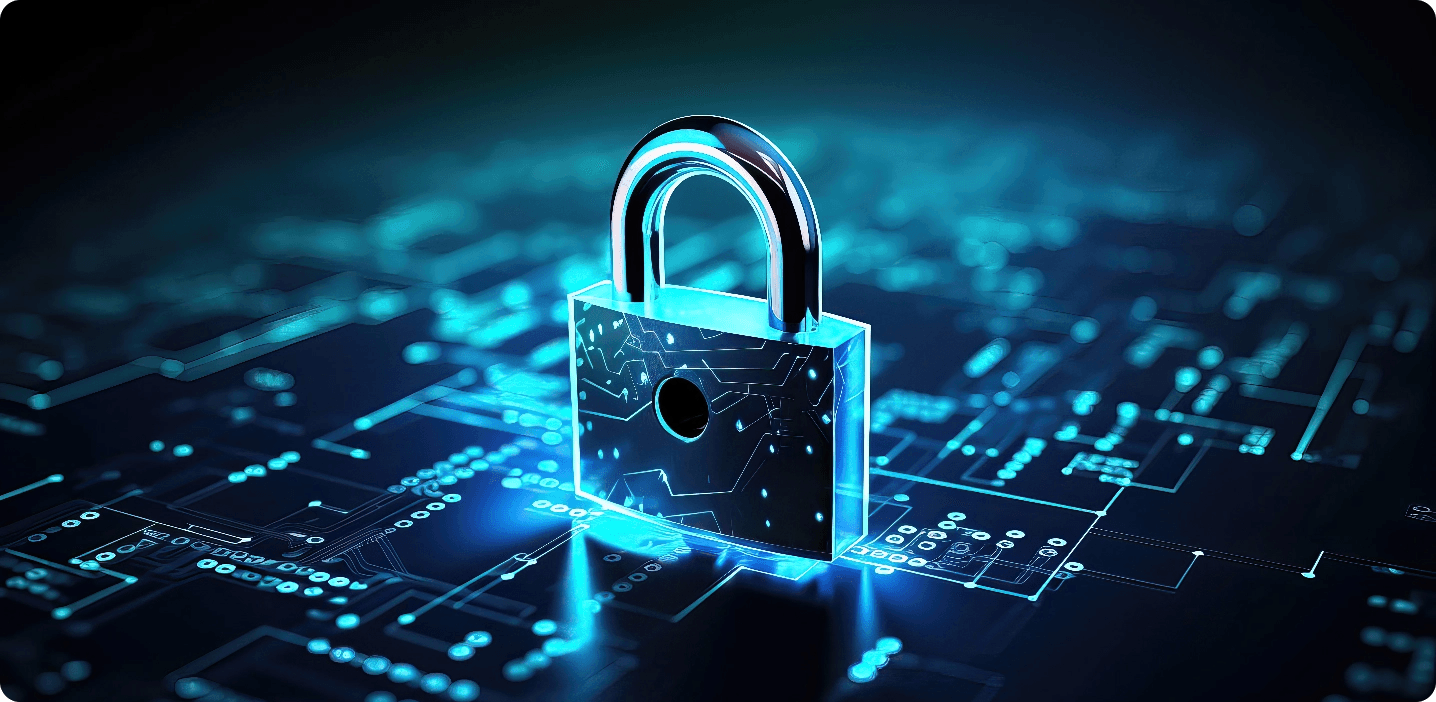
Conclusion
By integrating AI-driven anomaly detection with an intuitive alert management system and interactive visualization, financial institutions across banking, insurance, and retail industries significantly improved their fraud detection capabilities. Investigators were empowered with a deeper, more dynamic understanding of transaction behavior, allowing them to proactively target high-risk sectors with greater precision.
This case study highlights how leveraging AI can transform fraud detection, reducing workload while enhancing investigative accuracy and security.
For more information on implementing AI-driven fraud detection in your organization, contact us today!